Intel’s IPP is a cross-platform, free, vector and matrix library. It’s a great match for working with dsp and images in the JUCE framework, in particular on Windows.
IPP is cross-platform (happy on Linux and Windows) but only works on Intel Apple hardware. Apple has its own cross-architecture high performance library called Accelerate. Accelerate includes vDSP and vImage functions, and compatibility with Apple Silicon.
Let’s navigate the complexity and figure out how to reliably get fast vector and matrix math in our JUCE projects on Windows, including in CI.
Intel’s One API
Intel has something called “One API” — an ambiguous umbrella term for an insane amount of tooling, frameworks and APIs for data science, performance and machine learning.
Installing IPP (Intel Performance Primitives) and MKL (Math Kernel Library) are both possible through One API.
IPP or MKL?
JUCE has some loose support in the Projucer for including both IPP and MKL, and there’s been some confusion, as there’s overlap between the two.
Your choice on what to install and use depends completely on what functions you need access to.
In most cases, IPP is enough. It lets you do a lot with vectors, the same sorts of things that are in JUCE’s own FloatVectorOperations
.
MKL is…. more flexible and broad, although it does have some useful vector operations as well. Here’s a listing of what’s available in MKL’s vector math.
We’ll install both in this blog post, to make life flexible. Flexible is good.
JUCE Support
JUCE has some feature detection around parallel/sequential versions of IPP, but parallel is deprecated.
CMake support doesn’t really exist in JUCE, as of this post.
JUCE does provide FloatVectorOperations
as a wrapper around a subset of Apple’s Accelerate. However on Windows, JUCE sidesteps using IPP in favor of working directly with custom SSE vectorization.
In my opinion, vectorization would be more efficiently and comprehensively handled by IPP. There’s a much wider range of functions, with access to a lot of optimized high level functions. There’s currently an open Feature Request to ask JUCE to improve IPP support for this reason.
Installing IPP and MKL in CI
Although oneAPI is free to use, it’s not free to publicly distribute, which means the IPP package must be downloaded and installed on each CI run (if your code is public).
Although installation is kind of a mess, Intel kindly provides some examples for installing and using IPP in CI.
The examples contain the “offline installer” URLs we’ll want to use as well as the magic incantations to run the installer silently as a CLI — quite useful!
You can also find direct links to the “offline” installers over on Intel’s website.
As of 2023, Intel updates the library a couple times a year, so don’t be thrown off by IPP’s 2021.x
versioning scheme. I like to imagine they moved to a year-based release numbering strategy in 2021 and then changed their minds and made the extremely dubious decision to keep the 2021 prefix because…. of some esoteric compatibility thing that they just sweep under the rug? Oh, and as you’ll see later, CMake will report an entirely different version, such as 10.8
, just to make our life even spicier. This sort of inconsistency is unfortunately very typical of the “One API” ecosystem: it’s messy, bloated, and documentation is sketchy. The functions are great though! It’s just a bit wild west and needs some serious help on the DX/ergonomics side…
Choosing what to install from OneAPI
We’ll be using Intel’s bootstrapper.exe
on Windows to pick the components of OneAPI to install. You can see a list of the available components here.
We’ll stick to just installing IPP and MKL.
Here’s an example of silently running the full OneAPI installer and selecting IPP and MKL. You can run this locally to get setup:
# Download 3.5 gigs of Intel madness
curl --output oneapi.exe https://registrationcenter-download.intel.com/akdlm/irc_nas/19078/w_BaseKit_p_2023.0.0.25940_offline.exe
# Extract the bootstrapper
./oneapi.exe -s -x -f oneapi
# Silently install IPP and MKL, note the ":" separator
./oneapi/bootstrapper.exe -s -c --action install --components=intel.oneapi.win.ipp.devel:intel.oneapi.win.mkl.devel --eula=accept -p=NEED_VS2022_INTEGRATION=1 --log-dir=.
# List installed components
./oneapi/bootstrapper.exe --list-components
Note that Intel does tend break its urls over time, which makes it a bit annoying/brittle to use in CI. Perhaps this was just a 2023 thing, lets see if things stabilize in 2024.
You should see output that both IPP and MKL were installed and should be able to verify the intel libraries are now on disk:
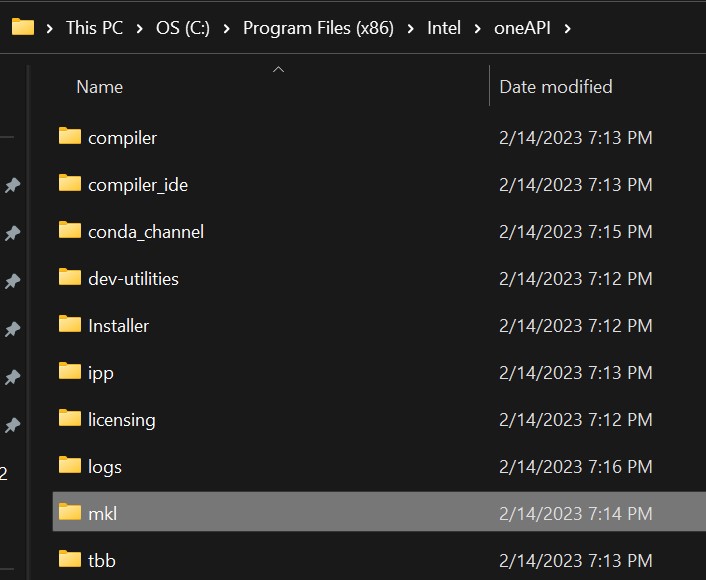
If you just need IPP, I highly recommend just grabbing their IPP offline installer, it’s only ~200MB.
If you’ve previously installed something from oneAPI, you might have to first run ./oneapi/bootstrapper.exe --action=remove
Caching the IPP install in CI
I highly recommend caching this install in CI vs. trying to bundle with your product, as the download is huge (~3.5 gigs). On GitHub Actions, the complete OneAPI download and install takes 25 minutes. IPP takes about 5-10 minutes. Restoring from cache takes about 10 seconds.
If working in GitHub actions, I recommend taking advantage of actions/cache/save
— the default actions/cache
will not save the cache if any step afterwards fails, which is pretty annoying for working on your CI pipeline.
Using actions/cache/save
allows you to just run the download and install once, no matter what happens afterwards.
Getting going with IPP and CMake
The fact that the One API is not open source (just free) certainly adds some friction with getting started (as well as filing bugs with Intel!)
However, Intel provides CMake helpers to help locate and install IPP. After installing on Windows, you can find them in C:\Program Files (x86)\Intel\oneAPI\ipp\latest\lib\cmake\ipp
Supposedly their CMake exports a variable called IPP_LIBRARIES
containing a list of targets you can link against, however of course it didn’t work for me. Nor others. Let me know if it worked for you.
However, you can still directly link individually exported targets to your target in CMake. All you need to do name them like so:
find_package(IPP)
if(IPP_FOUND)
target_link_libraries(MyProject PRIVATE IPP::ippcore IPP::ipps IPP::ippi IPP::ippcv)
endif()
A list of available IPP libraries can be found in that same CMake config. We’re detectives now!
ipp_iw,ippcore,ippcc,ippdc,ippch,ippcv,ippe,ippi,ipps,ippvm
The MKL CMake seems a bit more sophisticated and includes better output. There’s also a set of CMake OPTIONS
such as ENABLE_BLACS
. And they have an insane (in a bad way) “Link Advisor” to help you manually pick what to manually link against to.
In CMake you can link to MKL::MKL
, which is what I did for now. I only needed their vector math library (VML), but couldn’t figure out how to link against it directly.
Linkages, Initialization and CPU dispatching
So far, we’ve been proceeding with static linkage: the intel libraries are being built into your binary. Intel does support dynamic linkage as well, but I’m not clear when that would be a good decision.
The whole magic behind IPP is that it feature detects what CPU is in use at run time and picks the best primitive to use via its dispatching.
You used to have to call ippStaticInit
to setup the dispatcher, but no longer:
Since Intel Intel® IPP 9.0, there is no requirement to initialize the dispatcher by calling ippInit() for static linkage anymore.
See docs, assuming Intel didn’t break their web documentation again.
You can call the functions ippGetCpuType
and ippGetCpuFeatures
to confirm the dispatcher is happy.
The IPP Function Naming Shitshow
Both vDSP and Intel IPP have esoteric but (mostly) consistent naming practices. You’ll need to bring your decoder ring to the party to be able to parse them. Intel IPP in particular is cryptic as hell.
Let’s take a look at an example, ippiSet_8u_C1R_L
:
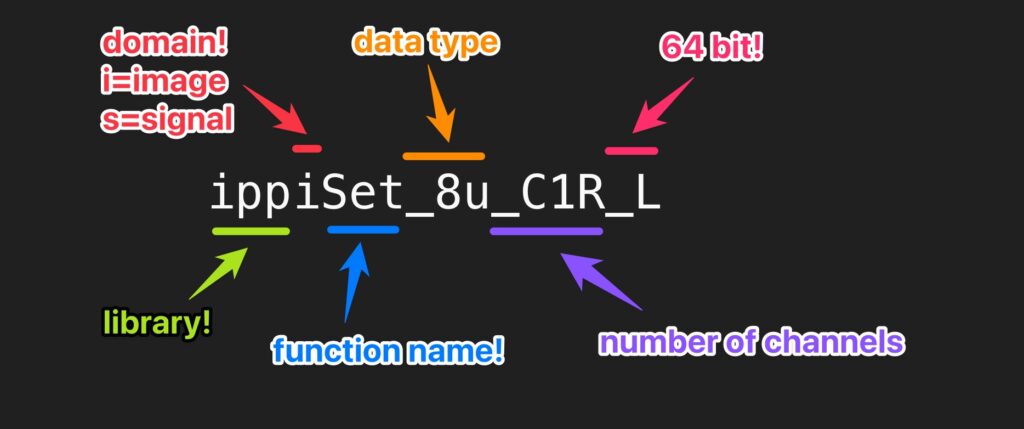
Yes, _L
means “platform aware” or basically “64 bit”. No, I don’t know what “L” stands for. No, don’t leave me a comment, I don’t want to know.
Moral of the story: If you want to do something simple, like fill a vector, you’ll need to visit the documentation for the root function name — like Set
— and perhaps whimper a quiet “what am I doing with my life?!” as you parse through 357 manifestations of the function call.
Intel does provide some docs around function naming but watch out! There are two identical looking links with completely different content! One for dsp and one for images.
It’s like programming before arguments were invented!
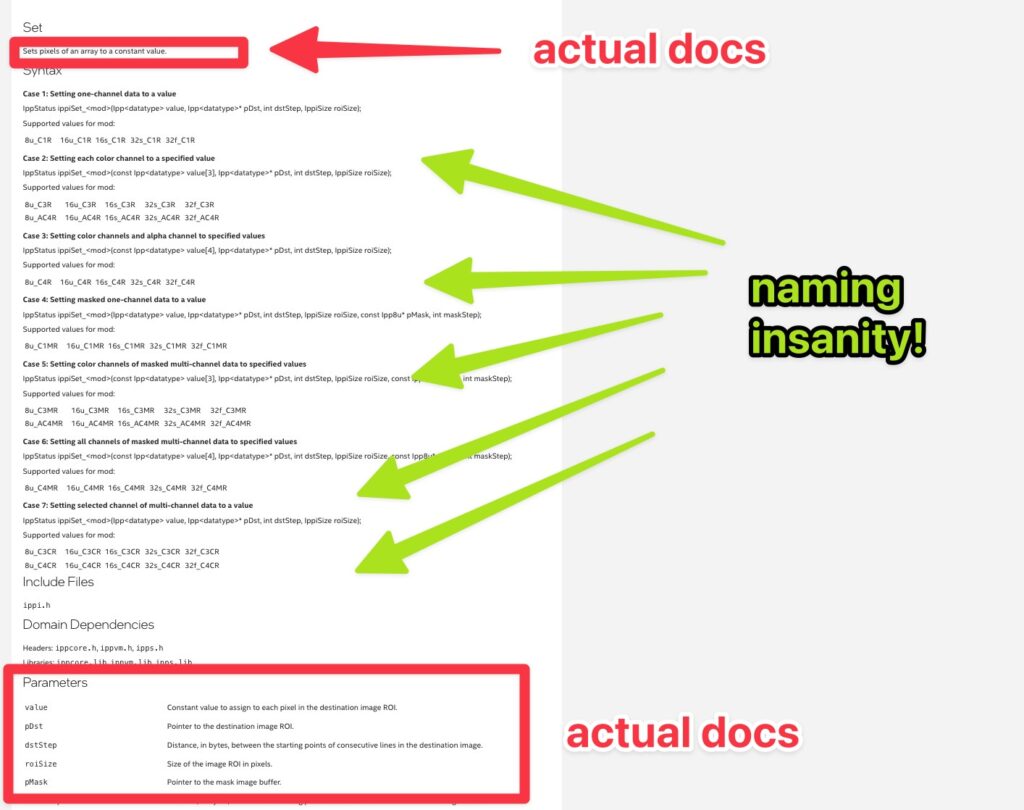
Luckily, you’ll quickly get the swing of things. Most of the variations are just for different data types, such as ippsSet32f
which is used with floats, ippsSet32s
for ints, etc.
Unlike vDSP, there are also specific “in place” descriptors. You’ll see many function variants with _I
appended to them, like ippsAdd_32f_I
These don’t take an output vector, as they are guaranteed to happily modify the input vector in place.
Be prepared to write spaghetti code
You’ll write the ugliest C++ code of your life in service to your new Intel overlords.
My suggestion: pretend you’re roleplaying as an 1980’s C programmer and marvel at how powerful these functions are. You’ll be happy to:
- Use a billion different custom versions of the
int
type for some reason - Call like 10 boilerplate functions in a row that all seem to take the same 6 arguments for some reason
- Initialize and pass “out” variables as arguments to acquire sizes for temporary buffers
- Allocate memory into those temp buffers with custom allocators.
- Don’t forget to call
ippsFree
!
Basically, it’ll feel like you’re writing half the damn library function yourself. However, the results will be worth it. Maybe I’m just spoiled from Apple’s Accelerate — while it’s almost as cryptic and sparsely documented, it doesn’t make you do the temporary buffer song and dance.
See a recent forum post of mine where I get a Gaussian blur working for an example of the beautiful poetry you are about to write.
A Running Example
You can see an example of IPP working with the JUCE framework and GitHub Actions in Pamplejuce, my JUCE template repo.
It downloads, installs, caches, tests and exposes the PAMPLEJUCE_IPP
and PAMPLEJUCE_MKL
preprocessor definitions when they are available.
Troubleshooting Intel IPP functions
What version are you on?
Watch out, because Intel will happily install multiple versions side by side. Your build system may cache older versions.
Save your sanity by inspecting the version:
auto version = ippsSetLibVersion();
What version of docs are you reading?
Intel seems to be the absolute worst at maintaining a simple docs website. It’s an impressive feat in 2023.
If you google “Intel IPP Set”, you are likely to land on an Intel documentation page from an outdated version. Which version? Who knows! But you can tell because of the yellow bar and the fact that HTML tags show up as plaintext in the html for some reason!
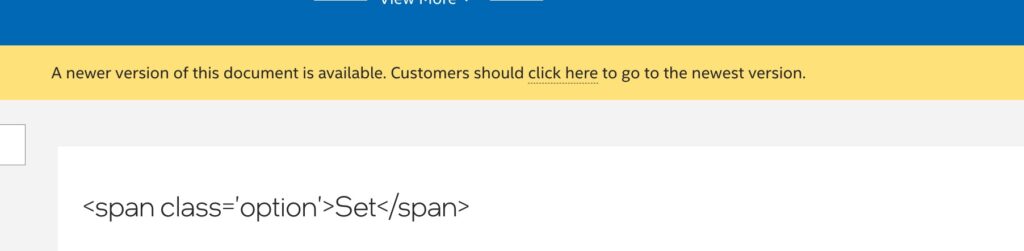
Clicking “click here” of course does not take you to the Set
function on the new docs site. Instead, it’ll take you to the root of their docs where you’ll have to use their search to find the function.
Translation: In 2023, you can’t actually google IPP function names. You’ll need to use Intel’s slow and shitty docs site search. Remember to use the root function name (ie, just Set
, not ippiSet
like it would appear in code)
Check the return status like it’s 1999
Most init
calls and function calls will return your new best friend, IppStatus
.
The docs pretend like you’ll get strings like ippStsNullPtrErr
from this status. No, sorry. You’ll get an int.
You’ll need to feed this global integer to ippGetStatusString
.
Here’s a table of status strings because yeah, you might want to just cut to the chase vs. build crufty error handling scaffolding everywhere.
Trial and Error
My experience is you’ll be lucky to get an error when things don’t work.
Instead, you are much more likely to get an Access Violation in the function and be stuck manually walking through and verifying each one of the repetitive pieces of setup code you had to write.
My experience is that when something isn’t working it’s usually a mental model issue. Like, I was wrong about how I thought the function worked or which data types I could use (which of course is the docs fault 🙂
Access violation reading location 0x00000000
Traditionally, this compiler error means an uninitialized pointer is trying to be dereferenced.
In the IPP context, especially when working with image and dsp buffers, this might happen behind the scenes in the library code. It probably means that either types are incorrect or a buffer wasn’t initialized. Comb through and make sure everything conceptually makes sense.
When working with image filters, make sure your types are aligned. For example, 32f kernel will not happily operate on a 8u image. You’ll need to convert the images manually first, otherwise you’ll see an access violation.
Beware of ChatGPT
ChatGPT can be great for learning. But it tends to fail at being useful on more esoteric APIs such as Intel IPP. There are little to no examples of working IPP usage in the wild on GitHub, forums, etc.
So at least as of 2023, it tends to invent or use deprecated function names and has 0 idea how to write or troubleshoot IPP code. Basically, ChatGPT + IntelPP is a recipe for being gaslit and frustrated — you’ve been warned!
Leave a Reply